Today I will talk about the NVIDIA h100 Architecture.
🏛 NVIDIA Hopper Architecture In-Depth
🏭 Manufacturing Process
🤑 Profit, Economy and Competition
📈 Projected Revenue
☁️ Cloud Offering
⛷️ Other Players
⚡️ Tesla and H100
🔌 Power Supply of the H100
🔢 FP8
🤯 If that doesn't give you FOMO, I'm not sure what will
Second Part…
🏛 NVIDIA Hopper Architecture In-Depth
The NVIDIA H100 GPU based on the new NVIDIA Hopper GPU architecture features multiple innovations:
New fourth-generation Tensor Cores perform faster matrix computations than ever before on an even broader array of AI and HPC tasks.
A new transformer engine enables H100 to deliver up to 9x faster AI training and up to 30x faster AI. inference speedups on large language models compared to the prior generation A100.
The new NVLink Network interconnect enables GPU-to-GPU communication among up to 256 GPUs across multiple compute nodes.
Secure MIG partitions the GPU into isolated, right-size instances to maximize quality of service (QoS) for smaller workloads.
Numerous other new architectural features enable many applications to attain up to 3x performance improvement.
The full implementation of the GH100 GPU includes the following units:
8 GPCs, 72 TPCs (9 TPCs/GPC), 2 SMs/TPC, 144 SMs per full GPU
128 FP32 CUDA Cores per SM, 18432 FP32 CUDA Cores per full GPU
4 fourth-generation Tensor Cores per SM, 576 per full GPU
6 HBM3 or HBM2e stacks, 12 512-bit memory controllers
60 MB L2 cache
Fourth-generation NVLink and PCIe Gen 5
Figure 3 shows a full GH100 GPU with 144 SMs. The H100 SXM5 GPU has 132 SMs, and the PCIe version has 114 SMs. The H100 GPUs are primarily built for executing data center and edge compute workloads for AI, HPC, and data analytics, but not graphics processing. Only two TPCs in both the SXM5 and PCIe H100 GPUs are graphics-capable (that is, they can run vertex, geometry, and pixel shaders).
🏭 Manufacturing Process
TSMC is the starting point.
They make chip of 4nm silicon wafers.
Then CoWoS Packaging with many layers of test.
NVIDIA sends the chips from TSMC to Foxconn to make H100 SXM modules.
Next it is shipped to Wistron that integrates 8 H100 SXM modules with 4 NVSwitches → cooling → testing → Delivering a completed H100 board.
🤑 Profit, Economy and Competition
The selling price is around $270,000.
If you accommodate the raw material cost (wafer cost, die cost, Bose Einstein D0, yield, HBM cost, CoW cost, WoS cost, substrate cost, package yield, ATE/SLT cost, heatsink/TIM, NVSwitch cost, power delivery cost, and baseboard cost) - $54K
Gross Profit is almost ~80% - removing the R&D cost. So Nvidia is using the FOMO of IT to print money.
📈 Projected Revenue
Fiscal revenue 2024: $57B
Fiscal revenue 2025: $78B
☁️ Cloud Offering
If you are looking for a cluster of H100 - the waiting time is Q1 2024 (best) to Q3 2024(worst)
AWS is charing 98.32 per hour on demand ie $12.29 per H100 GPU hour - if you include all the infrastructure cost, in 3 years they can break even 6x times.
But practically the companies are signing contract for 3 Year reserved cost that is $43.157 per hour ie $5.40 - then the huge corporate discount.From my sources the price ~ $4 per hour.
Coreweave, Lambda, and Oracle have a higher allocation priority from NVIDIA, which means they can provide better quality of service and GPU renting options.
⛷️ Other Players
H100 has ~2.4x to ~2.7x the performance of the A100 with Mosaic’s stack.
Intel gaudi3 suppresses the H100 in some of the tasks. But it's debatable because it's not a direct apple-to-apple comparison.
AMD MI300 is one of the strongest completions of H100 because its cheaper.
AWS has Trainium which has business demand.
Google’s TPUv5 is ramping up and they are building a strong ecosystem for LLM inference.
SambaNova’s new 8-chip architecture can reduce the price of LLM training and inference. They are claiming that 256,000 tokens of sequence length can be handled.
⚡️ Tesla and H100
It seems that Tesla will play a crucial role in H100. Elon Musk, who previously had ambitions with OpenAI, now has a similar drive with Tesla (now known as X.ai) to become one of the top AI companies globally.
Presently Alphabets Waymo has the most advanced self-driving capability. Furthermore, Tesla is nowhere to be seen in the generative AI world. And Tesla is not using video processing GPU devices on the car. But they have data - they call it “fleet scale auto labeling” - a 45-60 second log of dense sensor data, including video, inertial measurement unit (IMU) data, GPS, odometry, etc., and sends it to Tesla’s training servers.
Tesla's AI infrastructure is currently quite limited, with approximately 4,000 V100s and 16,000 A100s. This number is considerably smaller than that of other major tech companies like Microsoft and Meta, who possess over 100,000 GPUs and plan to double that number in the near future. The reason for Tesla's underwhelming AI infrastructure is partly due to delays with their in-house D1 training chip.
It appears that Tesla is entering the self-driving car market and is enlisting the help of GenAI to create simulations. To support this endeavor, Tesla is planning to acquire over 1,000 H100 HGX servers from Quanta Computer, as well as additional servers from SuperMicro throughout this year. By the end of the year, Tesla is expected to have 15,252 H100 GPUs at their disposal.
However, Tesla may not utilize the full power of the GPU. Instead, they may rent the GPUs to X.ai for the purpose of training GPT-5 or GPT-6. In short, Tesla is making a significant move in GenAI.
🔌 Power Supply of the H100
The Nvidia H100 has thermal design power of 700 watts (W).
As technology continues to progress, the power requirements for AI accelerators are also increasing. For example, the Nvidia H100 needs 700 watts (W), which is much higher than the most commonly used CPU in data centers, the Intel Skylake/Cascade Lake, which requires less than 200W. The next generation of chips will require even more power to handle increased computing demands. This means that rack-level power needs could be more than 200 kW, while conventional CPU server racks can only provide 15-20Kw.
It's great to see companies like Vicor adapting and thriving in changing times. In the past decade, they've gone from providing basic power components to creating advanced power solutions for data centers. They've even partnered with big names like Nvidia, Google, AMD, Cerebras, Tesla, and Intel to power their data centers with innovative rack-level power solutions and AI accelerators. Right now, Vicor has the technology to power the data center of H100, which has had a great impact on their share price.
🔢 FP8
FP8 is a natural progression for accelerating deep learning training inference beyond the 16-bit formats common in modern processors. In this paper we propose an 8-bit floating point (FP8) binary interchange format consisting of two encodings - E4M3 (4-bit exponent and 3-bit mantissa) and E5M2 (5-bit exponent and 2-bit mantissa).
H100 GPU introduced support for a new datatype, FP8 (8-bit floating point), enabling higher throughput of matrix multiplies and convolutions. In this example we will introduce the FP8 datatype and show how to use it with Transformer Engine.
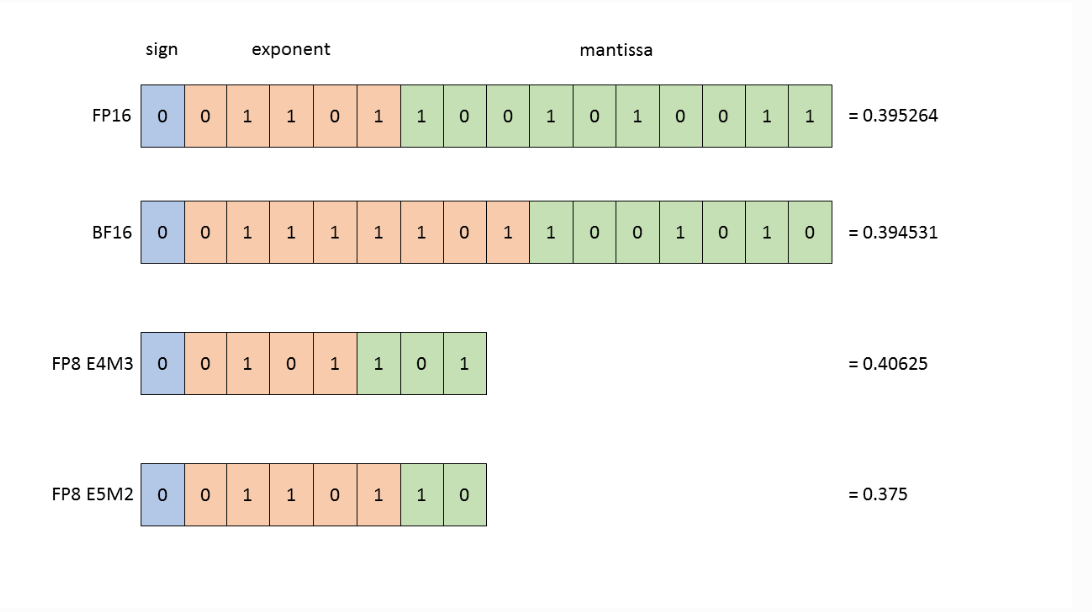
Mixed precision training - Need more space to discuss the idea. Will cover it in the second part of the series.
🤯 If that doesn't give you FOMO, I'm not sure what will
Meta is ordering a huge amount of GPUs that is well beyond their core recommendation engine needs because they want to get into generative AI. After all, if Zuck can light $15B a year on fire for the metaverse, why not $20B here for GenAI where they have a solid business case?
The industry will over-invest - because the search industry is in a transition phase after post-ChatGPT. Some killer applications on GenAI would make a great penny like Copilot, and Adobe Firefly, and justify the investment in GPUs.
The next leg has to be something around multi-modal LLM inference.
The industry is projecting that 2 million H100s will be shipped in the next Calnader year for Nvidia where the industry will train the base smaller model and llm inference.
The Second part
It will be continued - The second part will be on the performance of the H100.
**
I will publish the next Edition on Sunday and it will be available for the paid subscribers.
This is the 13th Edition, If you have any feedback please don’t hesitate to share it with me, And if you love my work, do share it with your colleagues.
It takes time to research and document it - Please be a paid subscriber and support my work.
Cheers!!
Raahul
**
This is AI generated right? Because some stuff is lifted from places I very clearly recognize it, some stuff wrong and seems LLM style hallucinated.